IFSR Newsletter 1994 Vol. 13 No. 1 March
Prof. Lofti A. Zadeh
University of California in Berkeley
(A conference speech held in St. Magdalena, Linz, Austria,summer 1993 on the occasion of a Conference on Fuzzy Logic, edited and summarized by Dr. Stephen Sokoloff).
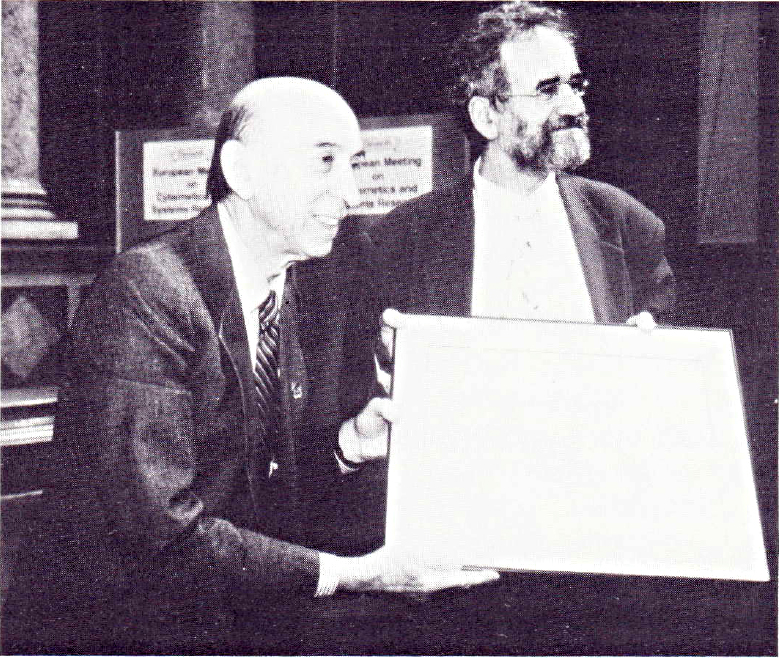
Prof. Lofti Zadeh (left) receiving an award from Prof. Robert Trappl at the EMCSR92 photo: Stephen Sokoloff, IFSR Newsletter 1993 Vol 13 No 1 (32)
Don’t waste your time trying to teach your computer how to park a car. Contemporary hardware-software systems can never learn to perform this task, since they are unable to cope with imprecision and uncertainty. Thanks to Lofti Zadeh and other visionary thinkers, however, the machines are now making rapid progress toward dealing with these factors.
Let’s begin by discussing the term machine IQ. There’s no precise definition for it yet, but we do have a vague feeling as to what we mean by it. The pace of progress toward enhancing the intelligence of machines began to pick up around 1990. I think that what happened is that up until then we had been, for the most part, using traditional computing, which is here referred to as hard computing. Around the beginning of the current decade, however, we began to use what might be called soft computing. In the former, rigor and precision are emphasized; one tries to minimize imprecision and uncertainty. In the latter, in contrast, we take the position that the real world is pervaded by imprecision and uncertainty, and we attempt to attain a tolerance for these in order to achieve tractability, robustness, high machine IQ, lower costs, and economy of communication.
Computer languages are maximally unambigous, but this is not the case with human languages, which assume a shared knowledge of context. For example, we say, “Meet me at five”, without specifying whether 5 AM or 5 PM is meant. That is why machines are often baffled by translation tasks.
The principle constituents of soft computing are fuzzy logic, neural network theory and probabilistic reasoning. The latter is concerned not only with probabilities but also with genetic algorithms, belief networks, etc. Soft computing therefore resembles a kind of a partnership in which various fields are involved. Fuzzy logic, for example, contributes a
method for dealing with imprecision.
There are some problems that can be very effectively solved with just one of the above techniques, but for others it is advantageous to employ a combination of them. Especially the utilization of fuzzy logic together with neural networks is becoming increasingly important. Particularly in Japan many neural-fuzzy consumer products are beginning to appear on the market.
Fuzzy logic is a term that has different meanings. lt was introduced by Lofti Zadeh in the early sixties [1]. ln the narrow sense it is the logic of approximate reasoning. In the broader sense, however, it is basically synonymous with fuzzy set theory, which includes fuzzy sets, fuzzy relations, fuzzy rules, etc. You can take practically any field and “fuzzify” it. Today the term “fuzzy logic” is mostly used in this latter, wider, manner.
By “fuzzifying” one gains greater generality, but one also exploits the tolerance for a lack of precision. It becomes apparent that humans can solve many problems because they are not precisely formulated. One example is the parking of a car, which actually involves three components: 1) finding a parking space, (2) deciding whether to park there (it might be too narrow or loo far away from your destination), and 3) maneuvering the car into it. Humans can accomplish these tasks because they are not exactly formulated, but machines cannot cope with them because of their inability to exploit the tolerance for imprecision. In the case of the first two, fuzzy logic solutions must be employed. These are basically human responses, expressed in the form of “if – then” rules. The third is reasonably well defined, however, and other techniques could conceivibly also be used to tackle it. Nevertheless that would involve
considerable difficulties because the problem has a high level of generality – it involves too many parameters. For that reason only the fuzzy logic approach has heretofore been attempted.
Linguistic variables play an important role in fuzzy logic; an entire range of values is thereby given a common label (eg. “small”, “medium”, “large”). In this way, data compression is achieved, since there are considerably fewer ranges than precise values. It is not possible within the framework of our newsletter to go into greater detail. The intention of this report is merely to convey an idea of what fuzzy logic is basically about and to give an impression of what can be accomplished by using it. Its applications are becoming increasingly important in industrial control processes and consumer products. One should be aware of the progress in this field.
[1] Zadeh L.A.: “Fuzzy sets. Information and Control ” vol. I (1965) pp. 338-353. The newsletter would like to thank Prof Dr. Erich P. Klement, organizer of the conference in Linz.